The global big data analytics market is projected to exceed $68B U.S. dollars by 2025 according to Statista. While you may have encountered the phrase “data analytics” or “big data” before, you may not be quite sure what these concepts are.
With that in mind, our experts created this immersive data analytics guide. Below, we discuss what data analytics is, why it is important, and how it can benefit large corporations. We’ll even outline how you can start your journey to a career in this exciting industry.
Table of Contents
What is Data Analytics, and why is it so relevant today?
Importance of Data Analytics for large corporations
Types of Data Analysis
What are the 5 steps of the Data Analysis process?
What skills are required to become a Data Analyst?
How do beginners learn Data Analytics?
What is Data Analytics and why is it so relevant today?
Before we dive too deep into our data analytics guide, it is important to define “data analytics.” Generally speaking, big data refers to massive data sets that are too complicated to be processed and analyzed via traditional applications.
Instead, analysts must use predictive tools and machine learning technologies to extract actionable insights from these data sets.
Data analytics are used for many purposes, such as digital marketing and project management. Businesses can also leverage data analytics to predict likely outcomes of major events that will impact their bottom line.
For instance, data analytics can help a corporation project how a new product will perform upon release.
Massive corporations are investing millions of dollars into the development of new products or services. This does not include the funds that they spend on marketing those products.
With so much at stake, the leaders of these companies want to ensure that they are making data-driven decisions. Data analytics can allow leaders to mitigate risks while also increasing their chances of achieving sustainable success in the competitive digital marketplace.
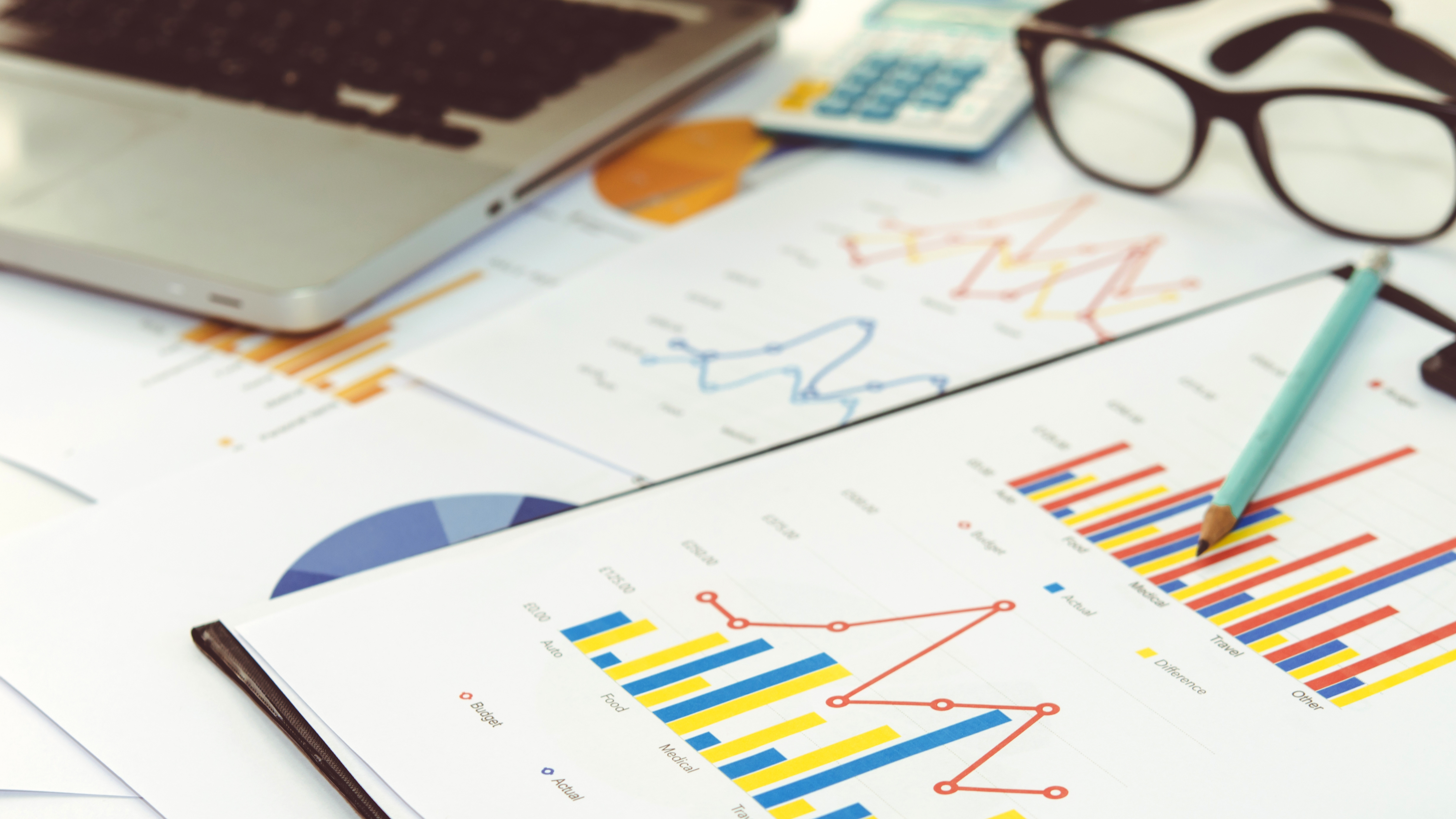
Importance of Data Analytics for large corporations
The growth of the big data analytics market projected by Statista reveals just how valuable this approach is too large corporations. Stakeholders and thought leaders understand that innovative analysis technologies have the potential to give them a competitive edge within their respective industries.
While big data analytics can produce many benefits for an organization, we have identified a few of the most notable below:
Optimized spending & cost reduction
Perhaps the biggest advantage of data analytics tools is that they help corporations to optimize spending and reduce costs. These technologies allow businesses to efficiently store, process, and analyze massive amounts of data. This can reveal valuable insights into ways that a company can refine its current business practices.
While the exact mechanism of cost savings will vary from industry to industry, data analytics can assist virtually any type of organization. That is why top companies like Spotify, Facebook, Netflix, Walmart, and Costco all utilize big data analytics technologies. These large corporations are constantly searching for ways to improve their profit margins while also eliminating wasteful spending.
Enhance efficiency
Data analytics tools can also improve the efficiency of day-to-day operations. Companies can assess feedback from customers or clients. They can then interpret this data to extract meaningful information regarding pain points, buying behaviors, and much more. Companies can then create more personalized services, products, and advertising content.
Data analytics can also free up employees to take on more dynamic tasks. Instead of manually sorting through data and identifying trends, employees can spend more time serving a company’s loyal clients.
Develop competitive pricing strategies
When a company is launching a new product or service, it is vital that they price it appropriately. If they price it too low, they will not have large enough margins to be profitable. Conversely, listing a price that is too high can hurt overall sales and may cause the product launch to fail entirely.
The most effective way to develop competitive pricing strategies is to utilize data analytics. Companies can track what their competitors are doing while also assessing customer demands. They can implement sound pricing that will appeal to their target market.
Improve sales
Data analytics can impact several key business processes, including pricing and marketing strategies. When all aspects of a corporation’s business model are optimized with data analytics, sales and profitability can be improved. Companies can also become more effective at connecting with consumers, which can foster a sense of loyalty to the company.
Fuel innovation
Large corporations must always seek to evolve and innovate. Otherwise, they will likely be overshadowed by competitors within their industry. With that in mind, data analytics is the most effective mechanism to fuel innovation.
By analyzing customer data, corporations can identify pain points and create products to fill those needs. They can also improve upon existing products or services in accordance with shifting consumer desires.
Manage brand reputation
Modern consumers have increasingly started to interact with businesses online. Many clients prefer this method of interaction when compared to more traditional methods, such as visiting a brick-and-mortar storefront or speaking with a staff member over the phone.
In light of this fact, it is vital that corporations provide users with an enjoyable online experience.
Big data analytics tools are capable of providing key insights into the user experience. Companies can leverage this functionality to determine what consumers are saying about their products or services. They can then adjust their online strategy to address customer grievances and provide a more enjoyable experience. This can enhance a brand’s reputation and help further the overall mission of the corporation.
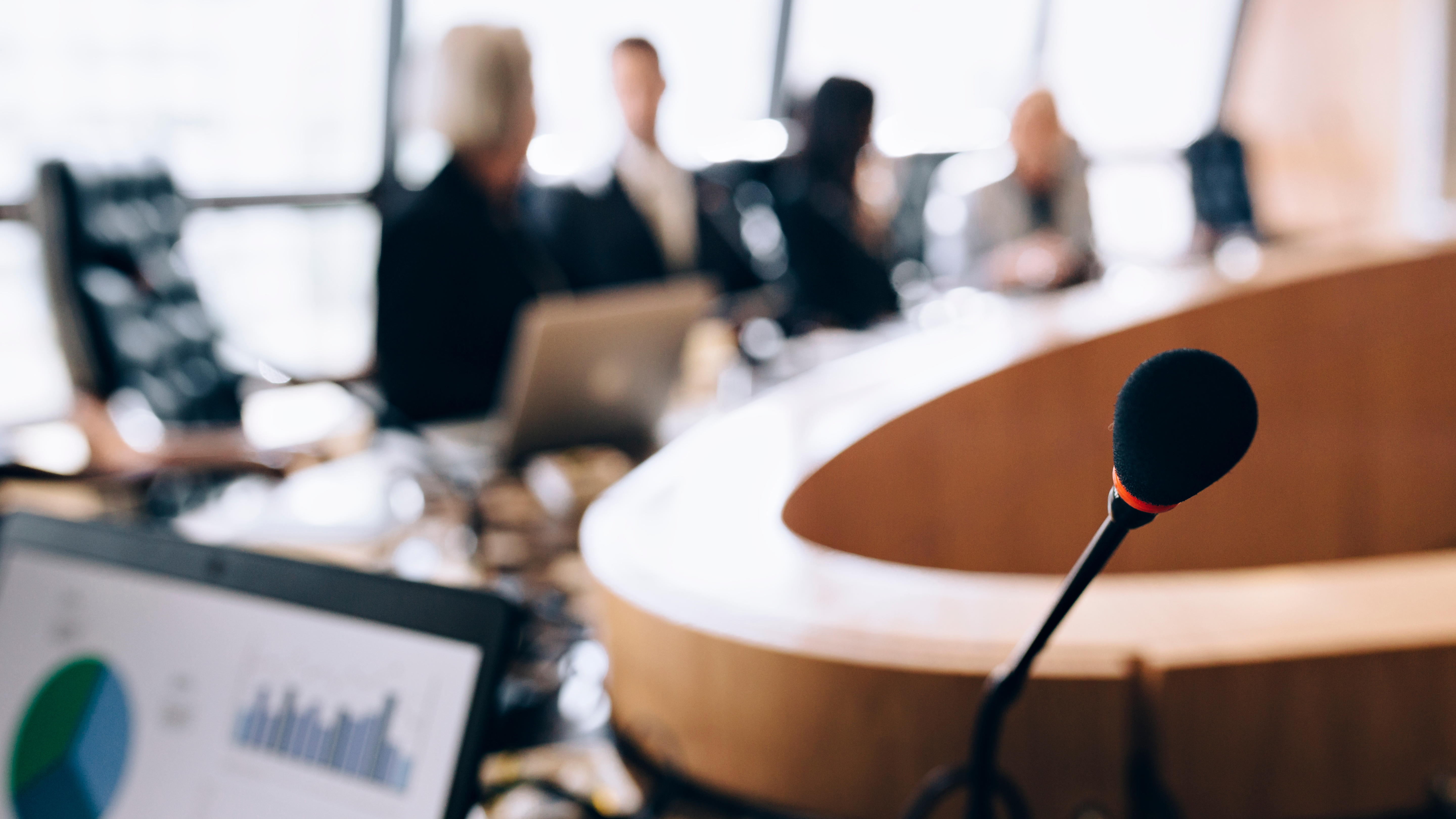
Types of Data Analysis
In the next part of our data analytics guide, we’ll shift our attention to the various methods of exploring these massive amounts of information.
There are four broad types of data analysis, which are as follows:
Descriptive Analysis
Descriptive analysis is one of the most commonly used forms of data analysis. As the name suggests, descriptive analysis is used to describe an event or events by providing a summary of past data.
Companies use descriptive analysis to determine “what happened.” However, the descriptive analysis does not reveal the “why,” which is an important distinction.
Descriptive analysis is usually interpreted and presented with visual charts. Visuals such as bar graphs, pie charts, and KPI dashboards are some of the most popular options used by businesses. Monthly revenue reports are another prime example.
Predictive Analysis
While determining what has already happened can provide some value to businesses, answering the question “What will likely happen next?” is far more important to stakeholders. That is where predictive analytics comes into play.
This type of data analysis utilizes a principle called “statistical modeling,” which requires innovative technologies and skilled human resources.
Predictive analysis is primarily used by large corporations. This is because it requires talented data analysts and vast technological resources. Predictive analysis can be used for sales forecasting, risk assessment purposes, and segmenting target audiences based on projected conversion rates.
Diagnostic Analysis
Typically, organizations will implement diagnostic analysis principles after using descriptive analysis. This is because diagnostic analysis seeks to reveal why something happened.
Using diagnostic analysis, businesses can zero in on the root cause of specific events or behaviors. Analysts identify correlations between events and then isolate factors that were causal (directly contributed) to a particular outcome.
By understanding both the “what” and “why,” corporations can attempt to duplicate successful events while avoiding undesirable outcomes.
Prescriptive Analysis
Prescriptive analysis is the newest field of data analytics. This practice relies heavily on machine learning and artificial intelligence (AI) algorithms to determine likely outcomes.
Prescriptive analysis not only forecasts what will most likely happen, but it also “prescribes” viable means of altering that outcome.
Thousands of companies would like to leverage prescriptive analytics, but few have the resources to do so. For now, only top corporations with massive budgets are able to use prescriptive analytics to guide their decision-making processes.
What are the 5 steps of the Data Analysis process?
The data analysis process for all four types of data analysis can be divided into 5 general steps. These stages are as follows:
Define the data that you need
Before an analyst begins harvesting data, it is vital that they determine which questions they want to answer. This will help them identify the type of data analysis that they will need to conduct. In addition, this will allow leaders to clearly define the objectives of their research.
For instance, if an analytics team wants to determine what their company’s turnover was last year, they will need to use descriptive analytics. However, if they are seeking to learn why employees are resigning, they will have to implement diagnostic analytical practices.
Once a team has identified the issue they want to resolve, analysts should create a list of questions that are relevant to that problem. Setting clearly defined goals will be essential when it comes time to actually start collecting data.
Data collection
Here is where things can get a bit tricky, depending on the problem that is being addressed.
Sometimes, data will be readily accessible, which will substantially streamline the data collection process. For example, a company’s HR department may conduct exit interviews with all outgoing employees. This means that analysts could review employee files and find out why they left — assuming they disclosed that information of course.
On the other hand, let’s say that the company does not conduct exit interviews or surveys. In this scenario, the analysts would have to reach out to past employees and find out why they left. Employees may not respond to attempts at contact, especially if they left on less-than-ideal terms.
After data analysts have collected as much relevant information as possible, they will be ready to move on to stage three of the process.
Data processing
Also known as “data cleaning,” data processing involves transforming the raw information into usable intelligence. Data cleaning is necessary because most data sets are flawed. They may contain missing values and other inconsistencies that can skew the end results.
Let’s stick with the example above and assume that the organization does not ask employees why they are resigning. If 20% of past employees that quit in the last year did not respond to inquiries about their resignation, this means that the analysts will face lots of missing values. They will need to clean this data up to account for the missing information.
Data analysis
Many people assume that data analysis is the final stage of the process. However, that is not the case.
During the data analysis phase, researchers look for relationships, hidden patterns, correlations, and other information that will provide valuable insight into the question at hand.
Data interpretation
This is where data analytics becomes valuable to corporations. Analysts must interpret the data and draw meaningful conclusions based on their findings. They may also convert these results into graphs, charts, and other formats that are easy to read.
The purpose of the data interpretation phase is to present the findings of the analysis to stakeholders in a compelling manner. Analysts should use the data to tell a story that resonates with decision-makers. This will ensure that their message hits home while also providing measurable benefits to the organization.
Skills required to become a Data Analyst
Data analysts are incredibly organized and talented individuals. They possess a diverse array of skills that aid them in every facet of the analysis process.
Data analysts must communicate with board members, executives, consumers, and virtually everyone in between. In addition, they have to be talented with innovative computer programs and information-gathering methods.
If you are interested in becoming a data analyst, you will need a great mix of soft skills and technical skills.
Soft skills
Soft skills directly impact how you work and interact with others. Soft skills are a bit more difficult to measure using standardized testing methods. That is why most employers still incorporate interviews into their hiring processes. Interviews allow managers to assess your soft skills firsthand and determine whether you are a good fit for their organization.
A few common examples of soft skills that data analysts need include:
Critical thinking ability
Data analysts are required to work through complex problems. Before they can start using data to accomplish this goal, they must first ask the right questions. That is where critical thinking comes into play.
Critical thinking skills allow individuals to identify which questions to ask and uncover connections that may not be immediately clear. Data analysts with strong critical thinking skills are able to stay grounded when tasked with solving difficult issues.
Communication and interpersonal skills
While assessing and interpreting data are some of the key responsibilities of a data analyst, it is only part of the equation. The best analysts in the world would not add value to their organizations unless they were able to effectively convey their findings.
As an analyst, you must be able to communicate using a variety of methods. This may include composing detailed emails, creating visually appealing charts, and presenting a verbal summary of your findings.
Strong presentation skills and confidence while engaging in public speaking are valuable skills for a data analyst.
Technical skills
Modern-day data analysts don’t rely on manual information-gathering practices. Instead, they are expected to use advanced computer technologies and software.
Data analysts must possess comprehensive technical skills so that they can effectively utilize these IT resources. A few of the most commonly used data analytics tools include:
SQL
SQL or Structured Query Language is one of the standardized programming languages. Data analysts used SQL to query and manage data that is held in databases. The SQL programming language is one of the best methods for collecting, storing, and organizing information obtained during data analysis.
SQL is widely considered to be the more advanced alternative to Microsoft Excel. SQL can handle massive data sets that exceed the capabilities of the spreadsheet program.
If you have an interest in becoming a data analyst or working with big data, then learning SQL is vital. Fortunately, it is one of the easier programming languages to learn.
Python or R programming knowledge
In addition to SQL, most data analysts use Python or R. These programming languages are used for statistical purposes. Python is one of the most popular languages for completing various tasks such as data cleaning, modeling, and crawling. It is also used for creating machine learning algorithms.
While Python is more widely used, R is a bit more useful for performing data visualization and statistical computing. With that being said, both languages are free and open-source, which means that you can start learning either with no upfront cost.
You will need to become a master of one of these two programming languages to become an analyst. Most of the time, employers do not have a preference. They are more concerned with accurate data analyses than with which programming language their staff members are using.
Experience with AI and machine learning tools
Prescriptive analysis is poised to become the future of data analytics. As advanced AI and machine learning technologies become more accessible, the demand for data analysts that can perform these types of services will continue to rise.
Even if you seek employment with a company that does not yet use prescriptive analysis, you will need to be well-versed in these tools.
A few of the most popular machine-learning platforms include Asana and Tableau. Asana is a mobile and web app that is designed to help companies manage their projects. Data analysts sometimes use Asana to manage and organize data.
Tableau Software produces technologies that are focused on business intelligence. Data analysts use Tableau to perform analysis and present their findings in an easy-to-understand visual format. It is one of the most popular data visualization technologies within the data analytics industry.
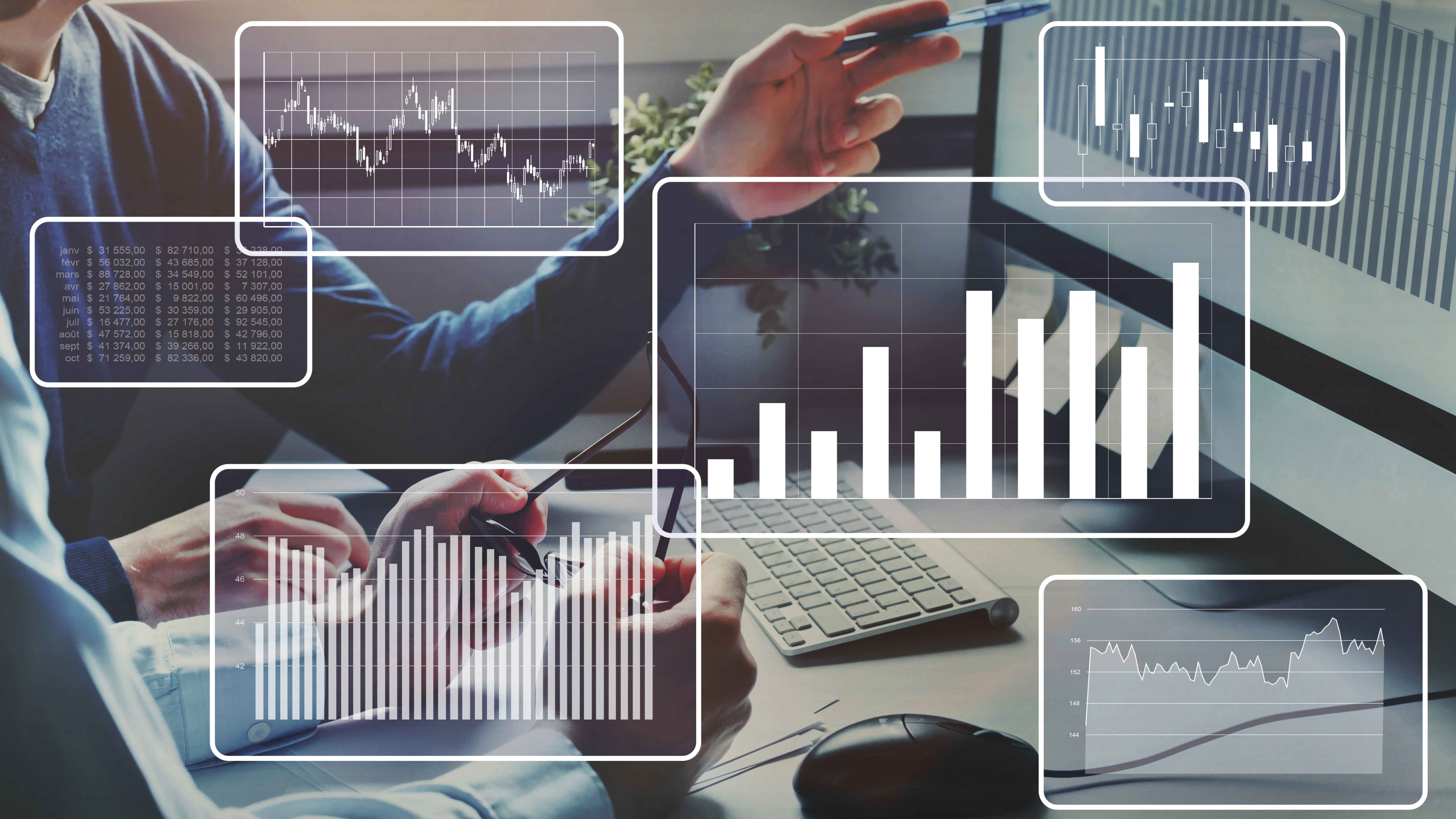
How do beginners learn Data Analytics?
As the digital marketplace becomes ever more competitive, the demand for data analysts will continue to surge. This exciting career field offers competitive salaries and plenty of opportunities for gainful employment with major organizations.
But how does a beginner become a talented data analyst? Read on to find out.
Pursue higher education
While not mandatory, many employers prefer to hire analysts with bachelor’s degrees in fields that relate to the profession. A few examples include mathematics, computer science, or statistics.
Learn core Data Analytics skills
For aspiring analysts that already have their degree or hope to circumvent the higher education route, we recommend learning core skills. This includes becoming familiar with SQL and either the Python or R programming language. Individuals should also work on their interpersonal skills to ensure that they are prepared for that first interview.
Consider Getting Certified
Specialized analytics certifications are a great way to demonstrate expertise with a particular program or software. These certificates can be obtained in addition to a college degree or as a standalone form of education.
Pathstream offers The Tableau Data Analytics Certificate, which is available online and can be completed in as little as 18 weeks.
Apply for Jobs
The next step is to apply for data analyst jobs. New analysts can go it alone or they can partner with a provider like Pathstream. We not only offer the various certification programs outlined above, but we also provide job placement assistance.
We connect our graduates with employers and provide ongoing career coaching to increase their chances of success.
Recapping our Data Analytics Guide
Has our data analytics guide inspired you to pursue a career in this exciting field? Would you like to start learning high-demand digital skills? Looking for a convenient platform that provides a flexible learning environment?
If you are interested in a career in data analytics, Pathstream can help you get started with our Tableau Data Analytics Certificate.
These programs include personalized support to help you succeed. Once you complete one of our online certification courses, you will be equipped with the skills you need to apply for a data analytics position with top companies.
Approximately 94% of our students get at least one job interview within 2 weeks of program completion. Our experienced staff will even help connect you with employers to ensure that you advance in your career quickly.
Contact us to learn more or sign up for one of our online certificate programs today!
Was this helpful?
Thanks! What made it helpful?
How could we improve this post?